微调ChatGPT-3.5模型训练后效果直逼GPT-4.0!如何使用AI大模型来fine-tuning(微调)训练自己私有知识库的机器人?
ChatGPT 3.5微调后的能力怎样?
ChatGPT3.5+微调+知识库=ChatGPT4
wowo!!!!!!!!!
当然!是在微调后垂直领域的发挥,通用模型老大还是GPT4!
今天 小智 分享下怎么来实现,快来一起看看吧。
(如果还有问题,或者想要联系智增增训练自己的知识库,欢迎联系我们定制需求,支持训练私有化大模型,支持定制各类AI系统:)
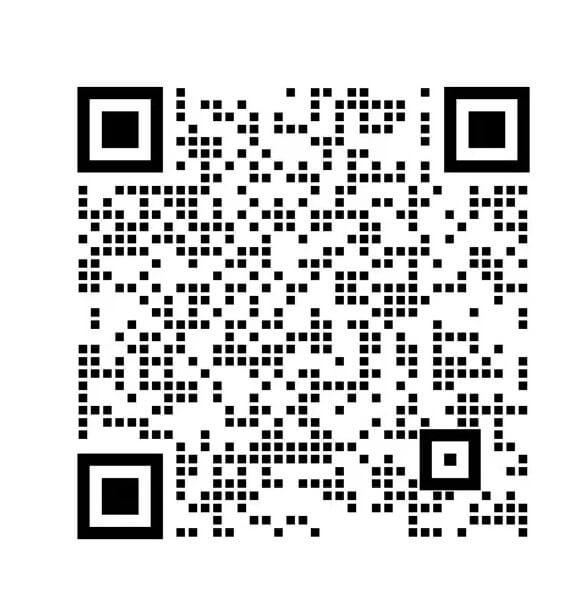
下面进入正文
这里主要是讲微调gpt-3.5的模型,使用自己企业的数据,在openai基础上训练一个自己行业的专有大模型
总的来说:
Fine Tuning的优点是,它能够使ChatGPT模型快速适应中文口语,并能够在短时间内实现中文口语的生成。此外,Fine Tuning技术也能够提高ChatGPT模型的准确性和效率,因为微调后的模型已经具有了更好的中文口语理解和生成能力。
Fine Tuning的缺点是,需要大量的中文口语数据集来进行微调。此外,微调后的模型可能会出现过拟合现象,导致对新数据的适应性不足。
微调(fine-tune)是什么?
网上内容多的是,不过多解释,只讲核心的
微调的基本思想是,先在大规模文本数据上预训练一个大型的语言模型,例如 GPT-3.5(这部分是大模型),然后使用特定任务的数据集(如法律、医疗),进一步对模型进行训练,以适应特定的任务(这部分是微调)。在这个过程中,模型的参数会进行微小的调整,使其在特定业务场景上的性能更好。
直接上代码:
1、首先需要拿到openai的key和url,
-
第1步:用手机号登录智增增,获取复制出key和url,地址:http://gpt.zhizengzeng.com/#/login
-
第2步:编写代码。注意配置的base_url是:
https://flag.smarttrot.com/v1
2、开始撸python代码:(其它语言类似)
- 准备训练数据
数据格式要注意形如下面:
{"messages": [{"role": "system", "content": "Marv is a factual chatbot that is also sarcastic."}, {"role": "user", "content": "What's the capital of France?"}, {"role": "assistant", "content": "Paris, as if everyone doesn't know that already."}]}
{"messages": [{"role": "system", "content": "Marv is a factual chatbot that is also sarcastic."}, {"role": "user", "content": "Who wrote 'Romeo and Juliet'?"}, {"role": "assistant", "content": "Oh, just some guy named William Shakespeare. Ever heard of him?"}]}
{"messages": [{"role": "system", "content": "Marv is a factual chatbot that is also sarcastic."}, {"role": "user", "content": "How far is the Moon from Earth?"}, {"role": "assistant", "content": "Around 384,400 kilometers. Give or take a few, like that really matters."}]}
下面是代码
API_SECRET_KEY = "你的智增增获取的api_key";
BASE_URL = "https://flag.smarttrot.com/v1"; #智增增的base_url
# files
def files():
openai.api_key = API_SECRET_KEY
openai.api_base = BASE_URL
resp = openai.File.create(
file=open("mydata.jsonl", "rb"),
purpose='fine-tune'
)
json_str = json.dumps(resp, ensure_ascii=False)
print(json_str)
- 上传训练数据
上传成功就自动开始训练了
API_SECRET_KEY = "你的智增增获取的api_key";
BASE_URL = "https://flag.smarttrot.com/v1"; #智增增的base_url
# jobs
def jobs(file_id):
openai.api_key = API_SECRET_KEY
openai.api_base = BASE_URL
resp = openai.FineTuningJob.create(training_file=file_id, model="gpt-3.5-turbo") #训练文件的id要从上一步获取得到
json_str = json.dumps(resp, ensure_ascii=False)
print(json_str)
- 检查是否训练完成
API_SECRET_KEY = "你的智增增获取的api_key";
BASE_URL = "https://flag.smarttrot.com/v1"; #智增增的base_url
# retrieve
def retrieve(ftid):
openai.api_key = API_SECRET_KEY
openai.api_base = BASE_URL
resp = openai.FineTuningJob.retrieve(ftid)
json_str = json.dumps(resp, ensure_ascii=False)
print(json_str)
- 使用微调模型
API_SECRET_KEY = "你的智增增获取的api_key";
BASE_URL = "https://flag.smarttrot.com/v1"; #智增增的base_url
# chat
def chat_completions(query):
openai.api_key = API_SECRET_KEY
openai.api_base = BASE_URL
resp = openai.ChatCompletion.create(
model="ft:gpt-3.5-turbo-0613xxxxxxxxxxxxxxxxxxx",
messages=[
{"role": "system", "content": "You are a helpful assistant."},
{"role": "user", "content": query}
]
)
json_str = json.dumps(resp, ensure_ascii=False)
print(json_str)
恭喜,大功告成!!
你就在gpt-3.5基础上训练了一个自己的模型了
© 版权声明
文章版权归作者所有,未经允许请勿转载。
相关文章
暂无评论...